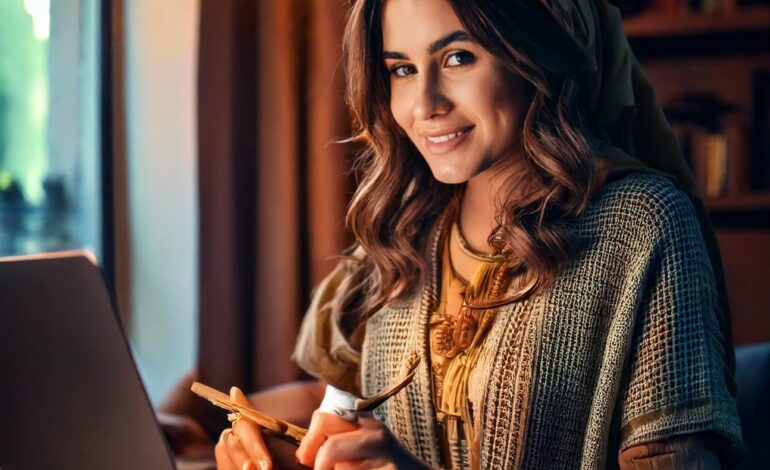
Get Started with Online Writing Jobs in Pakistan: Tips and Opportunities
Introduction
Online Writing Jobs As we all know, advanced techniques in machine learning have linearly increased over time in 2023 bettering performance in home usage. As prime techniques we can mention the following:
- Deep Learning: Applying neural networks with many layers to solve high dimensional data.
- Reinforcement Learning: Rewarding the model for making a suitable decision made when outputs are correct and doing the opposite when the output is wrong.
- Natural Language Processing (NLP): Assisting computer to arrange codes in a way that it tends to comprehend and produce human language reasonably close.
- Transfer Learning: Improving the performance of target tasks by incorporating information from previously learned tasks.
- Generative Adversarial Networks (GANs): Creating information, media, photos, and graphics with the help of two neural networks.
These approaches remain to enhance a plethora of industries by improving forecasting on demand and simplifying intricate processes.
The Evolution of Machine Learning Online Writing Jobs
Machine learning has a remarkable past, from being concepts on papers to physical functioning systems.
- 1950s: The concept of artificial intelligence began with the Turing Test by Alan Turing.
- 1960s: A cheap AI neural network was invented by Frank Rosenblatt and called the perceptron.
- 1970s-1980s: There was a decline in the AI research due to insufficient resources this was termed ‘AI winter’.
- 1990s: There was the emergence of a new generation of computer technology and development of better algorithms.
- 2000s: The growth in the volume of information led to the development of new technologies.
- 2010s: The popularity of deep learning approach has contributed to new developments in visual and audio understanding technologies.
The notion of machine learning has been changing and expanding the predicted impact on numerous domains.
Critical Components of Online Writing Jobs
Thus, machine learning models have a key set of components which are important for their operational development.
- Data Quality: Inadequate data can result in flawed models thus datasets with the utmost quality are required.
- Feature Engineering: Feature engineering entails designing and tweaking attributes and this is beneficial to understanding models.
- Algorithm Selection: Algorithm selection depends on the type of a problem and suitable techniques are picked from a neural network to linear regression.
- Model Evaluation: To gauge and alter models regarding performance and effectiveness, they can be measured through accuracy, precision and other metrics.
- Hyperparameter Tuning: Configuring learning rates and first or second order optimization in any setting improves the model.
- Regularization: Regularization aids addressing the problem of overfitting and helps generalize the model.
It is crucial to properly grasp and incorporate these components in order to develop effective machine learning models.
Current Cutting-Edge Machine Online Writing Jobs
Algorithms are crucial when it comes to machine learning and many institutions have managed to adopt superb Algorithms: while the foremost algorithms include:
Deep Neural Networks (DNNs):
- Application: Used in several fields such as Image and speech recognition, natural language processing
- Key Advantage: Best at complexity as an advantage in its use and application.
Convolutional Neural Networks (CNNs):
- Application: An application in the field of computer vision, primarily facial recognition.
- Key Advantage” In the processing image tasks, they are quite effective.
Recurrent Neural Networks (RNNs):
- Application: Time series analysis and modelling. This is language modelling
- Key Advantage: Best at capturing of patterns in sequential data.
Generative Adversarial Networks (GANs):
- Application: Data augmentation, image generation
- Key Advantage: Production of realistic artificial data
Reinforcement Learning (RL):
- Application: Robotics, gaming, autonomous systems
- Key Advantage: Optimal actions are learned via reward systems
Ethical Considerations and Challenges in Online Writing Jobs
There are many ethical issues in machine learning that need to be focused on including.
- Bias and Fairness: Algorithms need to be carefully designed to eliminate discrimination either against individuals based on race, gender or other such factors.
- Privacy Concerns: In order to function, systems based on machine learning require huge amounts of data which further complicates the issues of how data about individuals’ lives is accrued, preserved, and used.
- Transparency : The transparency of machine learning models and the decision making made by the models is an important concern as it affects trust and accountability.
- Security Risks: The protection of data from adversarial attacks and ensuring that data is honest is a huge obstacle.
- Regulatory Compliance: It is mandatory to keep to the necessary regulatory guidelines and rules in relation to ethical usage of machine learning technologies.
Future Trends in Machine Learning: What to Expect
Machine learning development does not stop, new trends are emerging and changing a large number of industries.
- Atoll: The spread of Atom out of its core userbase of advanced users exposes a wide network of people.
- XAI: XAI in a broader sense seeks to establish principles
- Reinforcement Learning: These are expected to deal with more complicated issues mainly in the fields of robotics and gaming.
- Edge Computing Integration: Alleviating the problem of latency by transferring the computing power to the edge devices.
- AI Ethics: It is increasingly essential to develop structures that will help address issues of bias in AI paradigms and promote fairness.
- Quantum ML: We expect that Quantum ML will enhance computation time far and even offer solutions to currently not solvable problems.
Conclusion and Final Thoughts on Online Writing Jobs
Indeed understanding advanced machine learning techniques is quite relevant for their absolute and every time fuller realization. Focusing on a machine learning professional, he needs to recognize a few of the facts that matter:
- Data Quality: This entails data of high quality that is ready by data cleaning, normalization and feature engineering.
- Hyperparameters: Refined methods of model parameters are sure to enhance performance.
- Ensemble: It means integration of more than one model to improve accuracy and robustness of and the model built.
The aim of continuous learning is specific success and any sort of professional sense in the world of technologies.